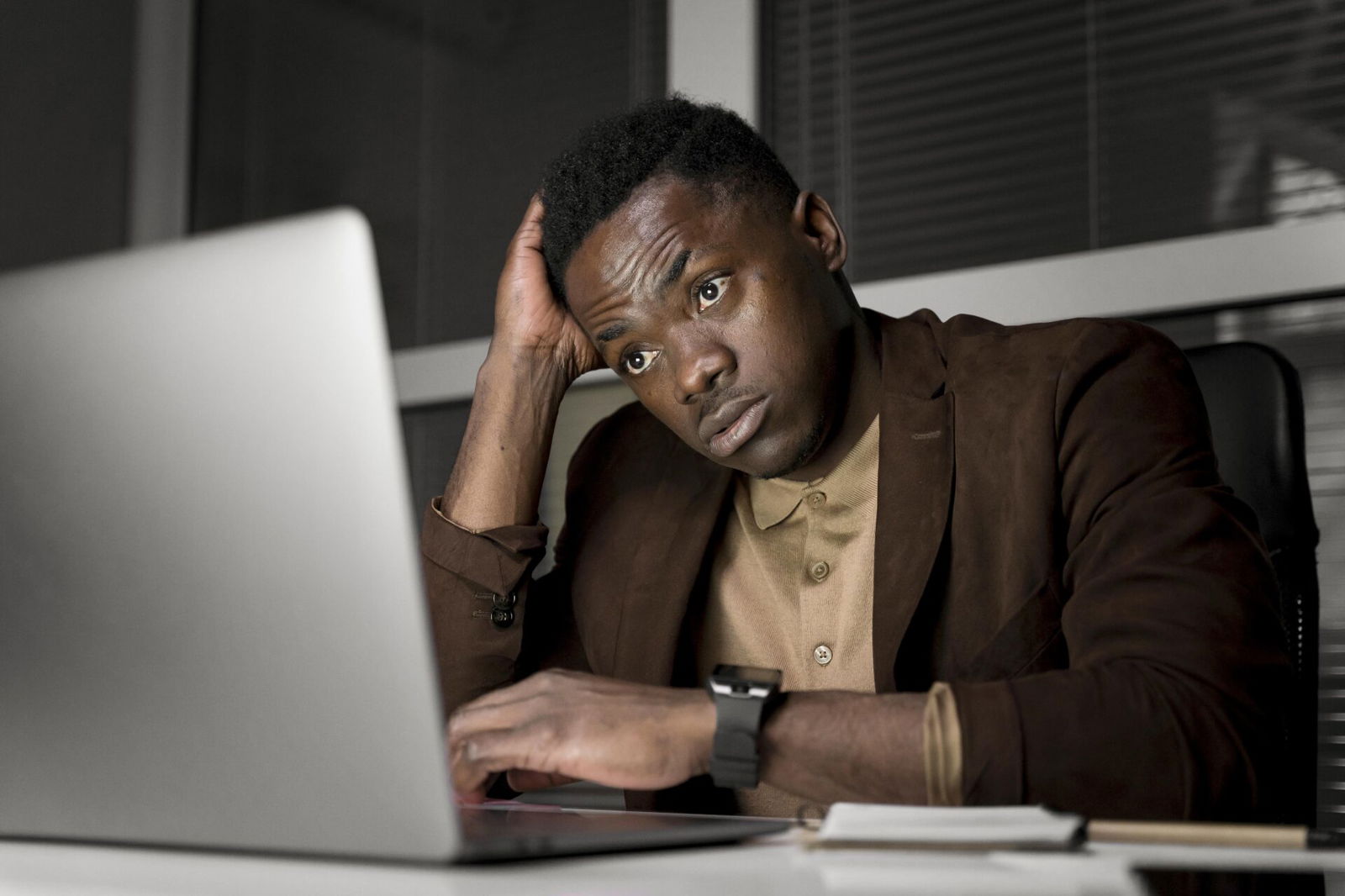
September 3, 2024
AI Shows Deep-Seated Bias Against African American Vernacular
A new study shows that large language models like ChatGPT have biases, including stereotypes against African American English speakers.
As more research about artificial intelligence explores the inner workings of the technology’s use of human language that has exploded following innovation from OpenAI and other players in the technology space, the anti-Black biases of these tools are being exposed.
According to a paper in Nature, large language models (LLM) like the ones used by Open AI’s ChatGPT program, operate with bias embedded in their programming. In the paper, the authors show that LLMs use dialect prejudice and hold raciolinguistic stereotypes regarding speakers who use African American English, Ars Technica reported.
According to the paper, “Dialect prejudice has the potential for harmful consequences: language models are more likely to suggest that speakers of AAE be assigned less-prestigious jobs, be convicted of crimes and be sentenced to death.”
Nicole Holliday, a linguist at the University of California, Berkeley, told Science.org that the findings of the paper deserve to be heard and intimately understood.
“Every single person working on generative AI needs to understand this paper;” Holliday also warned that although companies that make LLMs have attempted to address racial bias, “when the bias is covert…that’s something that they have not been able to check for.”
Despite efforts to fix the racial bias in these language models, the bias remains. The paper’s authors say that using human preference alignment to solve the problem of racial bias only serves to hide the racism that these models maintain inside their protocols.
According to the paper, “As the stakes of the decisions entrusted to language models rise, so does the concern that they mirror or even amplify human biases encoded in the data they were trained on, thereby perpetuating discrimination against racialized, gendered and other minoritized social groups.”
The paper goes on to tie together the potential prejudices of these LLM’s against AAE speakers with real-world examples of discrimination. “For example, researchers have previously found that landlords engage in housing discrimination based solely on the auditory profiles of speakers, with voices that sounded Black or Chicano being less likely to secure housing appointments in predominantly white locales than in mostly Black or Mexican American areas,” the report read.
According to the paper, “Our experiments show that these stereotypes are similar to the archaic human stereotypes about African Americans that existed before the civil rights movement, are even more negative than the most negative experimentally recorded human stereotypes about African Americans, and are both qualitatively and quantitatively different from the previously reported overt racial stereotypes in language models, indicating that they are a fundamentally different kind of bias.”
The paper also warned that, like American society becoming less overtly racist, the attitudes embedded in the subprocesses of artificial intelligence programs will allow for anti-Black racism to persist in more acceptable parameters as it relates to artificial intelligence.
The paper’s authors continued, “Worryingly, we also observe that larger language models and language models trained with HF exhibit stronger covert, but weaker overt, prejudice…There is therefore a realistic possibility that the allocational harms caused by dialect prejudice in language models will increase further in the future, perpetuating the racial discrimination experienced by generations of African Americans.”
RELATED CONTENT: Artificial Intelligence and Algorithms: 21st Century Tools for Racism